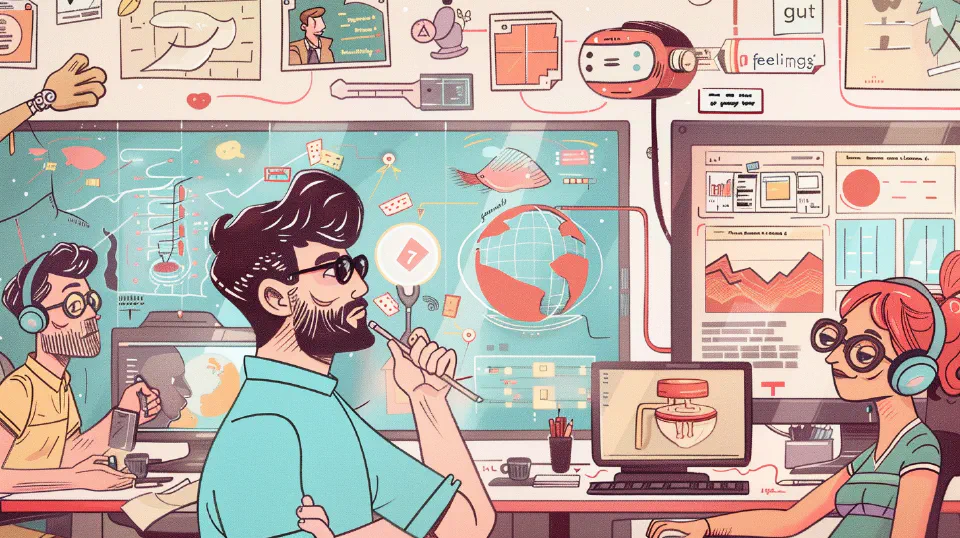
Why Data, Not Intuition, Is the Key to Unlocking Human Potential
In the realm of talent management and human resources, there's long been a romanticized notion of the intuitive leader who can spot potential at a glance or the HR professional with a gut feeling about a candidate. While intuition certainly has its place, the complexities of modern workplaces and the vast potential of human talent require a more sophisticated approach. Enter the era of data-driven talent management, where objective metrics, predictive analytics, and AI-powered insights are revolutionizing how we identify, develop, and unleash human potential.
The Intuition Trap
The allure of relying on gut feelings in talent management is understandable. It’s quick, it feels personal, and it taps into our human desire to trust our instincts. However, this approach is fraught with hidden biases and inconsistencies that can lead to missed opportunities and untapped potential.
Consider the case of Sarah, a mid-level manager at a tech firm. Her intuition told her that John, a quiet but diligent team member, wasn’t leadership material. However, when her company implemented a data-driven assessment program, it revealed that John possessed exceptional problem-solving skills and high emotional intelligence – key indicators of leadership potential that Sarah’s intuition had overlooked.
This scenario illustrates a crucial point: our intuitions, shaped by our experiences and biases, can often lead us astray when it comes to identifying and nurturing talent. In today’s diverse and rapidly evolving workplace, relying solely on gut feelings is akin to navigating a complex maze while blindfolded.
The Limitations of Human Intuition
To understand why data is superior to intuition in unlocking human potential, we must first recognize the limitations of our cognitive processes. Numerous studies in psychology and behavioral economics have identified a range of cognitive biases that affect our judgment, particularly in areas as complex as talent assessment.
One pervasive bias is the halo effect, where a person’s performance in one area influences our perception of their abilities in unrelated areas. For instance, a sales representative with excellent communication skills might be assumed to have good analytical abilities, even if there’s no evidence to support this.
Another significant bias is confirmation bias, where we tend to seek out information that confirms our pre-existing beliefs about a person’s potential. This can lead to a self-fulfilling prophecy, where our expectations shape a person’s performance rather than their true capabilities.
The inconsistency of intuitive judgments is another major limitation. Research has shown that even experienced professionals make significantly different decisions when presented with the same information at different times. This lack of consistency can lead to unfair and ineffective talent management practices.
Moreover, intuition-based approaches are difficult to scale. In small organizations, a leader’s intuition might be sufficient to identify and nurture talent. But as companies grow and become more complex, relying on the intuition of a few individuals becomes increasingly problematic.
The Power of Data in Talent Management
In contrast to the limitations of intuition, data-driven approaches offer a more objective, consistent, and scalable method for unlocking human potential. By leveraging vast amounts of information and sophisticated analytics, organizations can gain unprecedented insights into their talent pool.
Objective measurement of skills and potential is at the heart of data-driven talent management. Using a combination of assessments, performance metrics, and behavioral data, organizations can create comprehensive profiles of their employees’ capabilities. These profiles go far beyond traditional resumes or performance reviews, capturing nuanced information about an individual’s skills, working style, and potential for growth.
For example, Cognizant, a global IT services company, implemented a data-driven skills assessment platform that maps each employee’s capabilities across 1,300 different skills. This granular level of insight allows for precise matching of talent to projects and targeted development interventions.
Predictive analytics takes this a step further, using historical data and machine learning algorithms to forecast career trajectories and potential. These models can identify high-potential employees early in their careers, predict which employees are at risk of leaving, and even suggest optimal career paths based on an individual’s unique skill set and aspirations.
IBM’s Watson Career Coach is a prime example of how predictive analytics can be used to unlock human potential. The AI-powered system analyzes an employee’s skills, interests, and career history to provide personalized career advice and suggest relevant job opportunities within the company.
Personalized development plans based on data insights represent another powerful application of data in talent management. By analyzing an individual’s current skills, performance data, and career goals against the organization’s needs, data-driven systems can create tailored learning and development plans. These plans ensure that employees are developing the skills that are most relevant to their career aspirations and the organization’s strategic objectives.
Big Data and AI: The New Frontiers of Human Potential
As we move further into the digital age, big data and artificial intelligence are opening up new frontiers in talent management. These technologies are enabling organizations to process and analyze vast amounts of information, uncovering insights that would be impossible for humans to discern on their own.
Machine learning algorithms for talent identification are becoming increasingly sophisticated. These systems can analyze a wide range of data points – from an employee’s digital footprint to their collaboration patterns – to identify individuals with high potential for specific roles or leadership positions.
Google’s People Analytics team has been at the forefront of this approach. Their Project Oxygen used data from performance reviews, employee surveys, and other sources to identify the key behaviors of effective managers. This data-driven approach challenged many intuitive notions about leadership and has since been used to develop more effective management training programs.
Natural language processing (NLP) is another AI technology that’s revolutionizing talent management, particularly in the assessment of soft skills. NLP algorithms can analyze written communications, interview transcripts, and even social media posts to evaluate skills like communication, empathy, and cultural fit.
Unilever has implemented an AI-powered recruitment system that uses NLP to analyze video interviews. The system assesses candidates’ vocabulary, speech patterns, and facial expressions to evaluate their potential fit for various roles. This approach has not only increased the efficiency of Unilever’s recruitment process but has also helped to reduce unconscious bias in hiring decisions.
AI-driven career pathing and skill gap analysis are helping organizations to be more proactive in developing their talent. These systems can analyze an employee’s current skills against the projected needs of the organization, identifying potential skill gaps and suggesting learning opportunities to bridge these gaps.
Overcoming Resistance to Data-Driven Approaches
Despite the clear advantages of data-driven talent management, many organizations face resistance when implementing these approaches. Addressing privacy concerns is often the first hurdle. Employees may be uncomfortable with the idea of their every action being analyzed and used to make decisions about their careers.
To overcome this resistance, organizations need to be transparent about what data is being collected, how it’s being used, and what benefits it provides to employees. Implementing strong data protection measures and giving employees some control over their data can also help to build trust.
Balancing data with human judgment is another crucial aspect of successful data-driven talent management. While data provides valuable insights, it shouldn’t be seen as a replacement for human decision-making. Instead, data should be used to inform and enhance human judgment.
Spotify’s approach to performance reviews illustrates this balance well. The company uses a data-driven system to track employee performance and potential, but this data is used as a starting point for conversations between employees and their managers, not as the final word on performance.
Creating a data-positive culture in HR is essential for the success of data-driven talent management initiatives. This involves training HR professionals in data literacy, encouraging a mindset of continuous learning and experimentation, and celebrating success stories where data insights have led to better outcomes for employees and the organization.
Implementing a Data-Driven Talent Strategy
For organizations looking to leverage data to unlock human potential, implementing a comprehensive data-driven talent strategy is key. This process begins with building a robust talent analytics infrastructure. This involves not only implementing the right technologies but also ensuring that the organization has the capability to collect, process, and analyze relevant data.
Training HR professionals in data literacy is a crucial step in this process. HR teams need to be comfortable working with data, understanding statistical concepts, and interpreting analytical results. This doesn’t mean that every HR professional needs to become a data scientist, but they should be able to ask the right questions of data and use insights effectively in their decision-making.
Integrating data insights into daily talent management practices is where the rubber meets the road. This could involve using predictive analytics in succession planning, leveraging skill gap analyses in learning and development programs, or using real-time performance data in coaching conversations.
The Future of Data-Driven Talent Management
As we look to the future, several emerging trends promise to further revolutionize data-driven talent management. Continuous real-time assessment, enabled by advances in IoT and wearable technologies, will provide an even more granular and up-to-date picture of employee performance and potential.
Blockchain technology holds the potential to create verifiable, secure records of skills and achievements. This could lead to more accurate and trustworthy talent profiles, facilitating better matching of talent to opportunities across organizational boundaries.
Virtual and augmented reality technologies are opening up new possibilities for immersive skill evaluation. These technologies could enable more accurate assessment of complex skills in simulated environments, providing rich data on how individuals perform in realistic scenarios.
Conclusion: Embracing the Data Revolution in HR
The shift from intuition-based to data-driven talent management represents a significant competitive advantage for organizations. Those who can effectively leverage data to identify, develop, and deploy talent will be better positioned to innovate, adapt, and thrive in an increasingly complex business environment.
However, as we embrace this data revolution, we must not lose sight of the ethical considerations. The use of data in HR decisions must be fair, transparent, and respectful of individual privacy. Organizations need to develop clear ethical guidelines for the use of data in talent management and regularly audit their practices to ensure they’re living up to these standards.
Finally, while data is key to unlocking human potential, we must remember that talent management is ultimately about people. The goal of data-driven approaches should be to enhance, not replace, the human element in HR. By combining the objectivity and scalability of data with the empathy and judgment of skilled HR professionals, organizations can create truly effective talent management strategies that unlock the full potential of their workforce.
As we navigate this new era of data-driven talent management, one thing is clear: the organizations that can effectively harness the power of data while maintaining a human-centric approach will be best positioned to attract, develop, and retain the talent they need to succeed in the future of work.
Further Reading and Sources
-
“The Talent Delusion: Why Data, Not Intuition, Is the Key to Unlocking Human Potential” by Tomas Chamorro-Premuzic This book provides an in-depth exploration of how data-driven approaches can lead to better talent management decisions.
-
“People Analytics in the Era of Big Data: Changing the Way You Attract, Acquire, Develop, and Retain Talent” by Jean Paul Isson and Jesse S. Harriott A comprehensive guide to implementing data analytics in various aspects of HR and talent management.
-
Harvard Business Review - “21 HR Jobs of the Future”: https://hbr.org/2020/08/21-hr-jobs-of-the-future This article discusses how data and analytics are shaping new roles in HR, emphasizing the importance of data literacy in the field.
-
MIT Sloan Management Review - “With Goals, FAST Beats SMART”: https://sloanreview.mit.edu/article/with-goals-fast-beats-smart/ An exploration of how data-driven goal-setting can improve performance management.
-
“Artificial Intelligence for HR: Use AI to Support and Develop a Successful Workforce” by Ben Eubanks This book delves into how AI and machine learning are transforming various aspects of HR, including talent assessment and development.
-
Deloitte Insights - “2020 Global Human Capital Trends”: https://www2.deloitte.com/us/en/insights/focus/human-capital-trends.html Deloitte’s annual report provides insights into how data and analytics are shaping the future of work and talent management.
-
“The Future of Work: Attract New Talent, Build Better Leaders, and Create a Competitive Organization” by Jacob Morgan While not exclusively focused on data, this book provides context on how data-driven approaches fit into broader trends shaping the workplace.
-
Google re:Work - “Guide: Identify what makes a great manager”: https://rework.withgoogle.com/guides/managers-identify-what-makes-a-great-manager/steps/introduction/ This guide from Google explains how they used data to identify effective management behaviors in Project Oxygen.
-
“Predictive Analytics for Human Resources” by Jac Fitz-enz and John Mattox II A practical guide to implementing predictive analytics in HR functions.
-
World Economic Forum - “The Future of Jobs Report 2020”: http://www3.weforum.org/docs/WEF_Future_of_Jobs_2020.pdf This report provides valuable data on changing skill requirements and the increasing importance of data literacy across industries.
-
“The Employee Experience Advantage: How to Win the War for Talent by Giving Employees the Workspaces they Want, the Tools they Need, and a Culture They Can Celebrate” by Jacob Morgan This book includes insights on how data can be used to improve employee experience and unlock potential.
-
SHRM - “Developing a Data-Driven Culture”: https://www.shrm.org/resourcesandtools/hr-topics/technology/pages/developing-a-data-driven-culture.aspx This article provides practical advice for HR professionals looking to create a more data-oriented culture in their organizations.
-
“Data-Driven HR: How to Use Analytics and Metrics to Drive Performance” by Bernard Marr A comprehensive guide to leveraging data and analytics across various HR functions.
-
Journal of Applied Psychology - “Big Data Recommendations for Industrial-Organizational Psychology” This academic paper discusses how big data is changing the field of I/O psychology and its implications for talent management.
-
“Transformative HR: How Great Companies Use Evidence-Based Change for Sustainable Advantage” by John W. Boudreau and Ravin Jesuthasan This book provides case studies and strategies for using data to drive organizational change and unlock human potential.