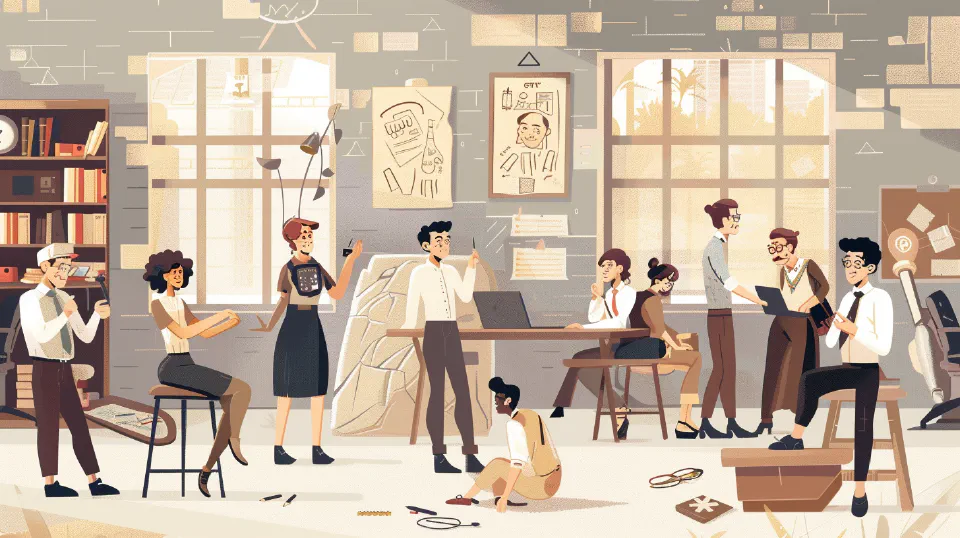
The Evolution of Skill Assessment: From Traditional Methods to AI-Powered Techniques
Skill assessment has undergone a remarkable transformation lately. From rudimentary paper-and-pencil tests to sophisticated artificial intelligence-driven platforms, the journey of skill assessment reflects the broader technological and cultural shifts in the workplace. This evolution has not only changed how organizations evaluate talent but has also redefined the very nature of skills in the modern workforce. As we delve into this fascinating progression, we'll explore the milestones, challenges, and innovations that have shaped the field of skill assessment, and examine how cutting-edge AI technologies are revolutionizing the way we identify, measure, and develop human potential.
The importance of effective skill assessment cannot be overstated in today’s rapidly changing business environment. As organizations grapple with digital transformation, evolving job roles, and the need for agile, adaptable workforces, the ability to accurately evaluate and predict skill proficiency has become a critical competitive advantage. This article will trace the historical development of skill assessment techniques, examine current best practices, and look ahead to the future of talent evaluation in an AI-driven world.
Throughout this exploration, we’ll consider not only the technological advancements that have driven change but also the shifting paradigms in our understanding of human potential, workplace performance, and the very nature of skills themselves. From the narrow focus on technical abilities to the growing recognition of emotional intelligence and adaptability as crucial workplace competencies, the story of skill assessment is also a story of our evolving comprehension of human capability and potential.
The Early Days: Traditional Skill Assessment Methods
The history of skill assessment dates back to ancient times, with early civilizations developing various methods to evaluate individuals’ abilities for specific roles. However, for our purposes, we’ll focus on the more recent history, starting from the early 20th century.
Paper-Based Tests and Interviews
In the early 1900s, skill assessment primarily relied on paper-based tests and structured interviews. These methods, while groundbreaking for their time, had significant limitations:
-
Standardized Tests: Organizations developed and administered standardized tests to evaluate specific skills or aptitudes. These tests often focused on cognitive abilities, basic mathematics, and language proficiency. The Army Alpha and Army Beta tests, developed during World War I to assess recruits, were early examples of large-scale standardized aptitude testing.
-
Structured Interviews: Hiring managers and HR professionals conducted face-to-face interviews using predetermined questions to assess candidates’ knowledge and experience. These interviews often followed a script or checklist to ensure consistency across candidates, but they were still subject to interviewer bias and inconsistency.
-
References and Recommendations: Employers relied heavily on personal and professional references to gauge a candidate’s skills and work ethic. While these could provide valuable insights, they were often subjective and could be influenced by personal relationships or limited exposure to the candidate’s full range of abilities.
While these methods provided some insights, they were often subjective, time-consuming, and limited in their ability to assess complex or soft skills. Moreover, they were prone to various biases, including the halo effect and confirmation bias, which could skew results and lead to poor hiring decisions.
The limitations of these early methods became increasingly apparent as the complexity of job roles grew and organizations began to recognize the importance of factors beyond technical skills. The need for more comprehensive and objective assessment techniques led to significant developments in the field of industrial-organizational psychology and the emergence of new assessment methodologies.
One of the major challenges of these early assessment methods was their inability to predict job performance accurately. Research in the mid-20th century began to show that the correlation between test scores or interview performance and actual on-the-job success was often weak. This realization spurred the development of more sophisticated assessment techniques that aimed to simulate real work scenarios and evaluate a broader range of competencies.
The Rise of Assessment Centers
The 1950s saw the emergence of assessment centers, particularly in military and government organizations. Assessment centers represented a significant leap forward in comprehensive skill evaluation:
-
Multi-method Approach: Assessment centers combined various evaluation techniques, including simulations, group exercises, and individual presentations. This multi-faceted approach allowed for a more holistic evaluation of candidates, assessing not just knowledge but also behavioral competencies and interpersonal skills.
-
Observable Behaviors: Assessors focused on observing and recording specific behaviors related to job performance, rather than relying solely on self-reported information. This shift towards behavioral assessment was influenced by the work of psychologists like B.F. Skinner and the growing field of behaviorism.
-
Multiple Assessors: The use of multiple trained assessors helped reduce individual bias and provide a more rounded evaluation of candidates. Assessors would typically undergo rigorous training to ensure consistency in their observations and ratings.
Assessment centers offered a more holistic view of a candidate’s abilities and potential. However, they were resource-intensive and typically reserved for high-level positions or large organizations with substantial HR budgets.
The development of assessment centers was largely driven by the needs of the military and large corporations. The Office of Strategic Services (OSS), the predecessor to the CIA, is often credited with developing one of the first comprehensive assessment centers during World War II to evaluate potential spies and operatives. This approach was later adopted and refined by companies like AT&T, which used assessment centers for managerial selection and development.
One of the key advantages of assessment centers was their ability to evaluate candidates in simulated work environments. For example, a candidate for a managerial position might be asked to lead a team through a problem-solving exercise, give a presentation on a business strategy, and handle a difficult employee in a role-play scenario. This approach provided insights into how candidates might perform in real-world situations, going beyond what could be gleaned from traditional interviews or tests.
Despite their effectiveness, assessment centers faced criticism for their high cost and time-intensive nature. They also raised questions about the validity of simulated environments and the potential for candidates to “perform” rather than demonstrate their natural behaviors. These challenges led to ongoing refinements in assessment center design and a push for more efficient, scalable assessment methods.
The Digital Revolution: Computer-Based Assessments
The advent of personal computers in the 1980s and 1990s ushered in a new era of skill assessment, bringing both opportunities and challenges.
Computer-Adaptive Testing (CAT)
Computer-adaptive testing represented a significant advancement in skill assessment technology:
-
Dynamic Difficulty Adjustment: CAT systems adjust the difficulty of questions based on the test-taker’s performance, providing a more accurate assessment of skill levels. This adaptive approach allows for a more precise measurement of ability across a wide range of skill levels, addressing the limitations of fixed-form tests that may be too easy or too difficult for some test-takers.
-
Efficiency: By tailoring the test to the individual, CAT can achieve accurate results with fewer questions, reducing testing time and fatigue. This efficiency makes it possible to assess a broader range of skills in a single testing session, providing a more comprehensive evaluation of a candidate’s abilities.
-
Immediate Scoring: Computer-based tests allowed for instant scoring and reporting, streamlining the assessment process. This rapid feedback enables quicker decision-making in hiring and development contexts and can provide immediate insights to test-takers about their performance.
The development of CAT was based on Item Response Theory (IRT), a psychometric approach that models the relationship between an individual’s ability and their responses to test items. This theoretical foundation allowed for the creation of large item banks and sophisticated algorithms to select the most informative questions for each test-taker.
One of the early successful implementations of CAT was the Graduate Management Admission Test (GMAT), which transitioned to a computer-adaptive format in 1997. This transition not only improved the test’s accuracy but also significantly reduced the time required for test administration, from about 4 hours to 2.5 hours.
However, early computer-based assessments were often limited to multiple-choice questions and struggled to evaluate complex problem-solving skills or soft skills effectively. The challenge of assessing higher-order thinking skills and practical abilities through computer-based methods remained a significant area of focus for researchers and test developers.
Online Assessments and Remote Testing
The rise of the internet in the late 1990s and early 2000s further transformed skill assessment:
-
Accessibility: Online assessments allowed organizations to evaluate candidates remotely, expanding the talent pool and reducing logistical barriers. This accessibility was particularly beneficial for global companies and organizations looking to tap into diverse talent markets.
-
Scalability: Web-based platforms made it easier to administer assessments to large numbers of candidates simultaneously. This scalability was crucial for organizations dealing with high volumes of applicants or conducting large-scale employee development initiatives.
-
Data Collection: Online systems facilitated the collection and analysis of assessment data, enabling organizations to identify trends and refine their evaluation processes. The ability to gather large datasets on test performance and outcomes paved the way for more sophisticated analytics and predictive modeling in skill assessment.
The transition to online assessments also introduced new possibilities for innovative question types and interactive elements. For example, multimedia-based questions, drag-and-drop interfaces, and simulations could now be incorporated into assessments, allowing for more engaging and realistic evaluation scenarios.
One of the significant advantages of online assessments was the ability to create and update test content more rapidly. This flexibility allowed organizations to keep their assessments aligned with rapidly changing skill requirements in various industries. It also facilitated the development of industry-specific or role-specific assessment tools that could be easily customized and deployed.
While online assessments offered numerous advantages, they also introduced new challenges, such as ensuring test security and preventing cheating in remote settings. Organizations had to develop sophisticated proctoring technologies and protocols to maintain the integrity of their assessments. This led to the emergence of online proctoring services that use a combination of human monitors and AI-powered systems to oversee remote test-taking.
The shift to online and remote testing also raised questions about the digital divide and equal access to technology. Organizations had to consider the potential for bias against candidates who might not have reliable internet access or up-to-date computer equipment. This concern led to efforts to develop mobile-friendly assessment platforms and to provide testing centers or equipment for candidates who needed them.
The Era of Big Data and Analytics
The 2010s saw a shift towards data-driven approaches in skill assessment, leveraging the power of big data and advanced analytics.
Predictive Analytics in Skill Assessment
Organizations began using historical data to develop predictive models for job performance:
-
Performance Correlation: By analyzing the relationship between assessment scores and actual job performance, companies could refine their evaluation criteria. This data-driven approach allowed organizations to identify which aspects of their assessments were most predictive of on-the-job success, leading to more focused and effective evaluation tools.
-
Talent Mapping: Advanced analytics enabled organizations to identify skill gaps and predict future talent needs more accurately. By combining assessment data with workforce planning models, companies could develop more strategic approaches to talent acquisition and development.
-
Customized Benchmarking: Data-driven approaches allowed for the creation of more nuanced, role-specific benchmarks for skill assessment. Rather than relying on generic industry standards, organizations could develop benchmarks based on the performance profiles of their top performers in specific roles.
The use of predictive analytics in skill assessment was greatly facilitated by advancements in machine learning and data processing capabilities. Organizations could now analyze vast amounts of data from various sources, including assessment results, performance reviews, productivity metrics, and even unstructured data like employee communications or project documentation.
One of the key challenges in implementing predictive analytics was ensuring the quality and relevance of the data used to build models. Organizations had to carefully consider issues of data bias, sample size, and the potential for overfitting models to historical data that might not reflect future skill needs. This led to the development of more sophisticated data validation techniques and the integration of external data sources to provide broader context for predictive models.
The rise of predictive analytics also sparked discussions about the ethical implications of using algorithmic decision-making in talent management. Questions arose about transparency, fairness, and the potential for perpetuating existing biases through data-driven models. These concerns led to increased focus on developing explainable AI models and implementing checks and balances to ensure equitable outcomes in skill assessment and talent decisions.
Behavioral Assessments and Personality Profiling
The field of skill assessment expanded to include more sophisticated evaluations of soft skills and personality traits:
-
Psychometric Tests: Advanced psychometric tools provided insights into candidates’ personality traits, work styles, and cultural fit. These assessments, based on psychological theories like the Five Factor Model of personality, aimed to predict how individuals might behave in various work situations and interact with others.
-
Situational Judgment Tests (SJTs): These assessments presented candidates with realistic workplace scenarios to evaluate their decision-making and problem-solving skills. SJTs became increasingly sophisticated, often incorporating multimedia elements and branching scenarios to provide more immersive and realistic assessment experiences.
-
Emotional Intelligence Assessments: Recognizing the importance of EQ in the workplace, organizations began incorporating emotional intelligence evaluations into their assessment processes. These tools aimed to measure abilities such as self-awareness, empathy, and social skills, which are crucial for effective leadership and teamwork.
The integration of behavioral and personality assessments into skill evaluation reflected a growing recognition of the importance of “soft skills” in the modern workplace. As automation and AI began to take over many routine tasks, uniquely human abilities like creativity, adaptability, and interpersonal skills became increasingly valuable.
One of the challenges in developing and implementing these assessments was ensuring their cultural relevance and fairness across diverse populations. Many early personality and behavioral assessments were developed primarily in Western contexts and needed to be carefully adapted and validated for use in global organizations. This led to increased research into cross-cultural psychology and efforts to develop more universally applicable assessment tools.
Another significant development in this area was the use of natural language processing and text analytics to infer personality traits and behavioral tendencies from candidates’ written responses or social media activity. While these approaches offered the potential for more naturalistic assessments, they also raised concerns about privacy and the ethical use of data in employment decisions.
The rise of behavioral and personality assessments also led to discussions about the stability of these traits over time and the extent to which they could be developed or changed. This sparked interest in growth mindset theories and the potential for using assessment results not just for selection decisions but also for personalized development plans and coaching initiatives.
While these advancements offered deeper insights into candidates’ potential, they also raised concerns about privacy and the ethical use of personal data in employment decisions. Organizations had to carefully balance the desire for comprehensive candidate information with respect for individual privacy and compliance with data protection regulations like GDPR.
The AI Revolution: Current Trends and Future Directions
The latest frontier in skill assessment leverages artificial intelligence and machine learning to create more sophisticated, adaptive, and comprehensive evaluation systems.
Natural Language Processing (NLP) in Skill Evaluation
AI-powered NLP technologies are transforming how we assess communication skills and analyze qualitative data:
-
Automated Essay Scoring: NLP algorithms can evaluate written responses for content, style, and coherence, providing objective assessments of writing skills. These systems are trained on large datasets of human-scored essays and can analyze factors such as argument structure, vocabulary usage, and grammatical complexity.
-
Sentiment Analysis: Advanced NLP tools can analyze the tone and emotional content of written or spoken responses, offering insights into a candidate’s communication style and emotional intelligence. This capability is particularly valuable for assessing customer service skills or evaluating leadership potential.
-
Resume Parsing: AI-driven resume analysis can quickly extract and categorize relevant skills and experiences from unstructured text, streamlining the initial screening process. These systems can also identify patterns and relationships between different skills and experiences, providing a more nuanced understanding of a candidate’s profile.
The development of more sophisticated NLP models, such as OpenAI’s GPT (Generative Pre-trained Transformer) series, has opened up new possibilities for natural language interaction in assessments. These models can engage in more human-like dialogue, potentially allowing for more dynamic and adaptive assessment experiences.
One of the challenges in implementing NLP-based assessments is ensuring fairness and avoiding bias, particularly in terms of language proficiency and cultural differences. Researchers and developers are working on techniques to make NLP models more culturally aware and capable of evaluating content rather than just language proficiency.
Another area of development is the use of NLP for analyzing verbal responses in video interviews or simulations. These systems can evaluate factors like speaking pace, vocabulary diversity, and even detect signs of nervousness or confidence. While promising, these applications raise important questions about privacy and the potential for bias in automated personality assessments.
Video-Based Assessments and Facial Recognition
AI technologies are enabling more nuanced evaluations of non-verbal communication and soft skills:
-
Facial Expression Analysis: AI algorithms can analyze facial expressions during video interviews to assess emotional responses and engagement levels. These systems are trained on large datasets of facial expressions and can detect subtle changes that might not be apparent to human observers.
-
Speech Pattern Analysis: Advanced speech recognition tools can evaluate factors such as speaking rate, tone, and fluency to assess communication skills. These analyses can provide insights into a candidate’s confidence, clarity of expression, and ability to modulate their communication style.
-
Body Language Interpretation: Some systems attempt to analyze posture and gestures to provide insights into a candidate’s confidence and interpersonal skills. This technology is still in its early stages but shows promise for providing a more comprehensive assessment of non-verbal communication.
The use of video-based assessments has grown significantly, particularly in the context of remote hiring and virtual interviews. These tools allow organizations to conduct asynchronous interviews, where candidates record responses to predefined questions, which are then analyzed by AI systems and reviewed by human evaluators.
One of the main advantages of video-based assessments is the ability to standardize the interview process and reduce the impact of interviewer bias. By having all candidates respond to the same questions under similar conditions, organizations can make more objective comparisons between candidates.
However, the use of facial recognition and body language analysis in hiring decisions has raised significant ethical concerns. Critics argue that these technologies may discriminate against candidates with disabilities, neurodivergent individuals, or those from cultural backgrounds with different norms of non-verbal communication. As a result, many organizations are approaching these technologies cautiously, often using them as supplementary tools rather than primary decision-making factors.
Another challenge in video-based assessments is ensuring a positive candidate experience. Some job seekers find one-way video interviews impersonal or anxiety-inducing. Organizations must balance the efficiency gains of these tools with the need to create a welcoming and engaging application process.
Research is ongoing to improve the accuracy and fairness of video-based assessments. This includes developing more diverse training datasets, incorporating cultural context into analysis algorithms, and creating more transparent evaluation processes that candidates can understand and trust.
Adaptive Learning Assessments
AI is powering a new generation of adaptive assessments that go beyond traditional computer-adaptive testing:
-
Real-Time Skill Mapping: AI systems can continuously update skill profiles based on performance in various tasks and simulations. This dynamic approach allows for a more nuanced and current understanding of an individual’s capabilities, reflecting the rapid pace of skill evolution in many industries.
-
Personalized Challenge Levels: Advanced algorithms can generate unique assessment paths for each individual, optimizing the evaluation process for accuracy and efficiency. These systems can quickly identify areas where a candidate excels or struggles, focusing the assessment on the most informative tasks for that individual.
-
Predictive Skill Development: By analyzing patterns in skill acquisition, AI systems can recommend personalized learning paths to address skill gaps and accelerate development. This integration of assessment and learning creates a continuous improvement cycle, transforming skill evaluation from a point-in-time event to an ongoing process.
Adaptive learning assessments are particularly valuable in fields with rapidly evolving skill requirements, such as technology and digital marketing. These systems can quickly incorporate new skills or competencies into the assessment framework, ensuring that evaluations remain relevant and forward-looking.
One of the key advantages of adaptive assessments is their ability to provide a more engaging and less stressful experience for test-takers. By adjusting difficulty levels in real-time, these systems can keep candidates challenged without overwhelming them, potentially leading to more accurate assessments of true capabilities.
However, the complexity of adaptive systems also presents challenges in terms of transparency and explainability. It can be difficult for candidates to understand how they are being evaluated when each person receives a unique set of questions or tasks. Organizations must work to create clear communication around the assessment process and provide meaningful feedback to candidates.
Research in this area is focusing on developing more sophisticated adaptive algorithms that can handle multidimensional skill assessments, incorporating both cognitive and non-cognitive factors. There’s also growing interest in using reinforcement learning techniques to continuously improve the assessment process based on outcomes and feedback.
Virtual Reality (VR) and Augmented Reality (AR) in Skill Assessment
Immersive technologies are opening up new frontiers in skill evaluation:
-
Realistic Simulations: VR environments allow for the assessment of skills in highly realistic, controlled scenarios that would be difficult or dangerous to replicate in the real world. For example, a VR simulation could assess a surgeon’s skills without risking patient safety, or evaluate a pilot’s decision-making under extreme conditions.
-
Stress Testing: VR simulations can evaluate how candidates perform under pressure or in complex, dynamic situations. This is particularly valuable for roles that require calm decision-making in high-stress environments, such as emergency responders or financial traders.
-
Spatial and Technical Skills: AR technologies enable the assessment of spatial reasoning and technical skills in interactive, three-dimensional environments. This can be especially useful for evaluating abilities in fields like architecture, engineering, or advanced manufacturing.
VR and AR assessments offer the potential for highly immersive and engaging evaluation experiences. They can provide a level of realism and interactivity that traditional assessments can’t match, potentially leading to more accurate predictions of on-the-job performance.
One of the challenges in implementing VR/AR assessments is the cost and technical requirements. High-quality VR systems can be expensive, and not all candidates may have access to the necessary equipment. Some organizations are addressing this by setting up dedicated assessment centers with VR capabilities, while others are exploring more accessible AR solutions that can work with smartphones or tablets.
Another consideration is the potential for VR sickness or discomfort, which could affect assessment results. Developers are working on techniques to minimize these issues, such as reducing motion sickness triggers and allowing for frequent breaks in longer simulations.
Research in this area is exploring the use of VR/AR for assessing soft skills and interpersonal abilities. For instance, VR simulations can place candidates in complex social scenarios, such as difficult customer interactions or team conflicts, allowing for the evaluation of emotional intelligence and conflict resolution skills in a controlled yet realistic environment.
As VR and AR technologies become more sophisticated and accessible, they are likely to play an increasingly important role in comprehensive skill assessment. The challenge for organizations will be to integrate these immersive assessments into their broader talent management strategies, ensuring that the insights gained translate into meaningful hiring and development decisions.
Challenges and Considerations in Modern Skill Assessment
While AI and advanced technologies offer tremendous potential, they also present new challenges that organizations must navigate:
-
Ethical Considerations: The use of AI in skill assessment raises important questions about privacy, consent, and the potential for algorithmic bias. Organizations must carefully consider the ethical implications of collecting and analyzing extensive personal data, including biometric information from video assessments or physiological responses in VR simulations.
There’s also the risk of perpetuating or amplifying existing biases through AI algorithms. If training data reflects historical biases in hiring or promotion decisions, AI systems may reproduce these biases in their assessments. Organizations need to implement rigorous bias detection and mitigation strategies, including regular audits of assessment outcomes across different demographic groups.
Another ethical concern is the potential for AI assessments to infringe on candidate privacy. The use of social media data or extensive personality profiling may cross ethical boundaries, even if technically legal. Organizations must balance the desire for comprehensive candidate insights with respect for individual privacy and autonomy.
-
Data Security: As skill assessments collect increasingly sensitive data, ensuring the security and proper use of this information becomes critical. Organizations must implement robust data protection measures to safeguard assessment data from breaches or unauthorized access.
This includes not only technical security measures but also clear policies on data retention, use, and sharing. Candidates should be informed about how their data will be used and given options to control or delete their information where possible.
The global nature of many organizations adds complexity to data security considerations. Different regions have varying data protection regulations, such as GDPR in Europe or CCPA in California. Organizations need to ensure their assessment practices comply with all relevant legal requirements across their areas of operation.
-
Validity and Reliability: Organizations must rigorously validate AI-driven assessment tools to ensure they accurately predict job performance and don’t perpetuate existing biases. This involves ongoing studies to correlate assessment results with actual job performance, as well as regular reviews to ensure the assessments remain relevant as job roles evolve.
The rapid pace of technological change can make it challenging to establish long-term validity for new assessment methods. What works well today may become obsolete as job requirements shift. Organizations need to build flexibility into their validation processes, allowing for continuous refinement and updating of assessment criteria.
There’s also the challenge of establishing validity across diverse populations. An assessment that accurately predicts performance in one cultural context may not be equally valid in another. This necessitates cross-cultural validation studies and potentially the development of culturally specific assessment versions.
-
Human Oversight: While AI can enhance the assessment process, human judgment remains crucial in interpreting results and making final decisions. Organizations need to strike a balance between leveraging the efficiency and insights of AI systems and maintaining human involvement in critical talent decisions.
This involves training HR professionals and hiring managers to effectively interpret AI-generated assessment data, understanding both its potential and limitations. It also requires clear processes for when and how human judgment should override algorithmic recommendations.
There’s also a need for ongoing human oversight of AI systems themselves, monitoring for unexpected behaviors or outcomes that might indicate problems with the algorithms or training data.
-
Continuous Adaptation: As job roles and required skills evolve rapidly, assessment systems must be flexible enough to adapt to changing needs. This requires a commitment to ongoing research and development, as well as close collaboration between HR, business leaders, and technology experts to anticipate and respond to emerging skill requirements.
Organizations need to build agility into their assessment frameworks, allowing for the quick incorporation of new skill evaluations or the modification of existing ones. This might involve modular assessment designs that can be easily updated or AI systems capable of learning and adapting to new job requirements in real-time.
There’s also the challenge of balancing stability and change in assessment practices. While it’s important to keep assessments current, frequent changes can make it difficult to compare results over time or across different parts of the organization. Organizations need strategies for managing this tension, perhaps through core assessments that remain stable, supplemented by more flexible, role-specific evaluations.
By acknowledging and addressing these challenges, HR professionals can develop more robust and effective gamification strategies that deliver lasting value to both employees and the organization.
The Future of Skill Assessment
As we look to the future, several trends are likely to shape the evolution of skill assessment:
-
Holistic Evaluation: Future assessment systems will likely take a more holistic approach, considering not just skills and knowledge but also adaptability, learning potential, and cultural fit. This trend recognizes that in a rapidly changing work environment, the ability to learn and adapt may be more valuable than current skill proficiency in many roles.
We may see the development of more sophisticated models that integrate cognitive abilities, personality traits, values, and motivations into a comprehensive profile of individual potential. These models could help organizations make more nuanced decisions about not just job fit, but also long-term development potential and leadership capabilities.
There’s also growing interest in assessing collective intelligence and team dynamics. Future systems might evaluate not just individual skills, but how well a candidate’s profile complements and enhances existing team capabilities.
-
Continuous Assessment: Rather than point-in-time evaluations, organizations may move towards continuous skill assessment, leveraging data from ongoing work performance and learning activities. This shift recognizes that skills are not static and that regular, job-embedded assessment can provide a more accurate and current picture of capabilities.
We might see the rise of “invisible” assessments that seamlessly integrate into daily work processes, collecting data on performance, problem-solving approaches, and collaboration patterns. AI systems could analyze this data to provide ongoing insights into skill development and identify areas for improvement or redeployment.
This continuous approach could also enable more dynamic talent allocation, allowing organizations to quickly identify and deploy the right skills for emerging projects or challenges.
-
Integration with Learning Platforms: Skill assessment will likely become more tightly integrated with learning management systems, creating a seamless cycle of evaluation and development. As soon as a skill gap is identified, personalized learning recommendations could be automatically generated and delivered.
We may see the emergence of AI-driven “learning companions” that use ongoing skill assessments to guide individual development journeys, adapting learning content and experiences based on real-time performance data and changing job requirements.
This integration could also facilitate more effective knowledge sharing within organizations, with assessment systems identifying experts in specific areas and facilitating connections for mentoring or collaborative learning.
-
Blockchain for Skill Verification: Blockchain technology may be used to create secure, verifiable records of skills and certifications, streamlining the assessment process. This could lead to the development of “skill passports” that individuals carry throughout their careers, with verified records of assessments, experiences, and achievements.
Such a system could reduce reliance on self-reported skills or traditional resumes, providing a more reliable and comprehensive view of an individual’s capabilities. It could also facilitate more fluid movement between organizations, as skill verifications would be easily transferable and trustworthy.
We might see the emergence of decentralized skill marketplaces, where individuals can showcase their verified skills and organizations can find talent based on highly specific and authenticated skill profiles.
-
Neuroscience-Based Assessments: Advances in neuroscience may lead to new methods of assessing cognitive abilities and potential, possibly including brain-computer interfaces. While still in early stages, technologies like EEG-based assessments or fMRI scans could provide insights into cognitive processes, learning potential, and decision-making styles.
These technologies might offer more objective measures of abilities like multitasking capacity, stress resilience, or creative thinking. However, their use would likely be limited to specific high-stakes roles due to cost and ethical considerations.
We may also see the development of less invasive neurotechnology-based assessments, such as advanced eye-tracking or micro-expression analysis, that could provide deeper insights into cognitive processes and emotional responses during problem-solving or decision-making tasks.
As these trends unfold, HR professionals will need to stay informed and adaptable, continuously evolving their gamification strategies to leverage new technologies and meet changing employee expectations. The future of skill assessment promises to be more personalized, continuous, and integrated into the fabric of work itself, offering unprecedented insights into human potential and performance.
Conclusion
The evolution of skill assessment from traditional methods to AI-powered techniques represents a remarkable journey of innovation and adaptation. As organizations strive to identify, develop, and retain top talent in an increasingly complex and fast-paced business environment, the importance of accurate and comprehensive skill assessment has never been greater.
While AI and advanced technologies offer exciting possibilities for enhancing the accuracy, efficiency, and scope of skill evaluations, it’s crucial to approach these innovations thoughtfully. Organizations must balance the potential benefits of new assessment technologies with ethical considerations, ensuring that their practices are fair, transparent, and respectful of individual privacy.
As we move forward, the most successful skill assessment strategies will likely be those that combine the analytical power of AI with human insight and judgment. By embracing innovation while staying grounded in sound ethical principles, organizations can unlock the full potential of their workforce and drive sustainable success in the ever-evolving world of work.
The future of skill assessment is not just about measuring what individuals can do today, but about understanding and nurturing their potential for tomorrow. As assessment technologies continue to evolve, they have the power to transform how we perceive and develop human potential, creating more dynamic, adaptive, and fulfilling work environments for all.
Further Reading and Sources
-
“The Cambridge Handbook of Technology and Employee Behavior” edited by Richard N. Landers This comprehensive handbook provides in-depth coverage of how technology is changing workplace behaviors and assessment practices.
-
“Artificial Intelligence for HR: Use AI to Support and Develop a Successful Workforce” by Ben Eubanks Eubanks offers practical insights into how AI can be applied in various HR functions, including skill assessment and talent development.
-
“The Future of Recruitment: Using Artificial Intelligence to Enhance the Candidate Experience” by Swati Mishra This book explores how AI is transforming recruitment processes, including innovative approaches to skill assessment.
-
Society for Industrial and Organizational Psychology (SIOP) - White Paper on AI in Talent Assessment: https://www.siop.org/Research-Publications/Items-of-Interest/ArtMID/19366/ArticleID/3361/White-Paper-Artificial-Intelligence-in-Talent-Assessment This white paper provides a comprehensive overview of the current state and future directions of AI in talent assessment.
-
Harvard Business Review - “AI Can Help Assess Job Candidates — When It’s Used Wisely”: https://hbr.org/2022/06/ai-can-help-assess-job-candidates-when-its-used-wisely This article discusses the potential benefits and pitfalls of using AI in candidate assessment, offering practical advice for implementation.
-
World Economic Forum - “The Future of Jobs Report 2020”: http://www3.weforum.org/docs/WEF_Future_of_Jobs_2020.pdf This report provides insights into changing skill requirements and the implications for assessment and development strategies.
-
MIT Sloan Management Review - “The Future of Performance Management”: https://sloanreview.mit.edu/article/the-future-of-performance-management/ This article explores how technology is reshaping performance management practices, including ongoing skill assessment.
-
Journal of Applied Psychology - “The Validity and Utility of Selection Methods in Personnel Psychology: Practical and Theoretical Implications of 100 Years of Research Findings” This seminal paper provides a comprehensive review of the effectiveness of various selection methods, including skill assessments.
-
AI & Society - “Ethical Implications of Using AI in Talent Assessment” This academic article delves into the ethical considerations surrounding AI-powered assessment techniques.
-
Annual Review of Organizational Psychology and Organizational Behavior - “The Digitization of Employee Assessment” This review article provides a thorough examination of how digital technologies are transforming employee assessment practices.
-
“Handbook of Employee Selection” edited by James L. Farr and Nancy T. Tippins This comprehensive handbook covers various aspects of employee selection, including modern approaches to skill assessment.
-
“The Age of AI: And Our Human Future” by Henry A. Kissinger, Eric Schmidt, and Daniel Huttenlocher While not specifically focused on HR, this book provides valuable insights into how AI is reshaping various aspects of society, including the workplace.
-
Journal of Business and Psychology - “Game-Based Assessment: The Use of Video Games for Talent Selection and Development” This academic article explores the potential of using video games and gamification in skill assessment and talent development.
-
“Data-Driven HR: How to Use Analytics and Metrics to Drive Performance” by Bernard Marr Marr’s book provides practical guidance on leveraging data and analytics in various HR functions, including skill assessment.
-
Gartner Research - “Emerging Technologies and Trends Impact Radar: Artificial Intelligence in HR” This report offers insights into how various AI technologies are likely to impact HR practices, including skill assessment, in the coming years.