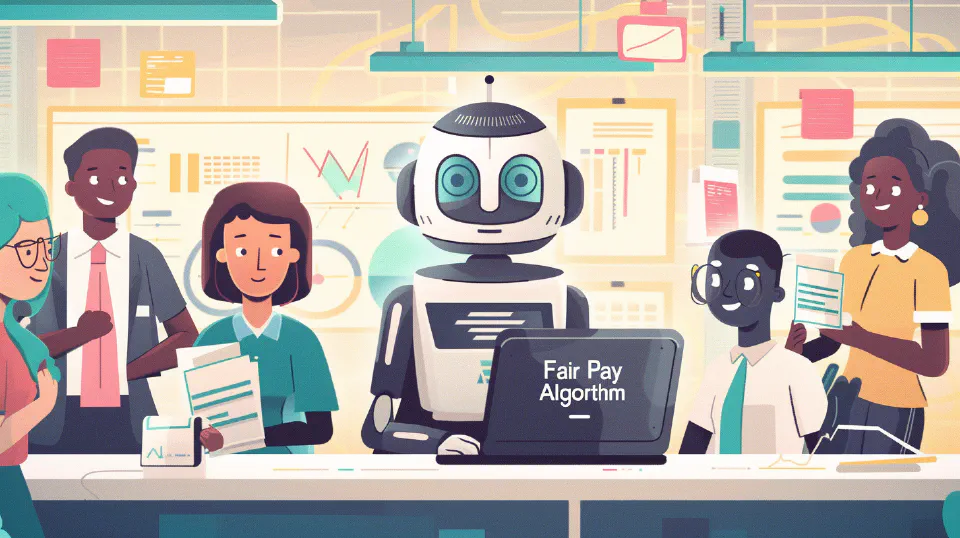
AI-Driven Compensation: Using Machine Learning for Fair Pay Practices
Artificial Intelligence (AI) and Machine Learning are revolutionizing how organizations approach compensation strategies. This technological advancement promises to address long-standing issues in pay equity, bias elimination, and fair compensation practices. By leveraging vast amounts of data and sophisticated algorithms, AI-driven compensation systems are enabling HR professionals to make more informed, objective, and fair decisions about employee pay. This article explores the transformative potential of AI in compensation management, examining how it works, the key issues it can solve, and practical steps for implementation. As we delve into this innovative approach, we'll uncover how AI is not just a tool for efficiency, but a powerful ally in creating more equitable and competitive compensation structures that benefit both employees and organizations.
The Current Compensation Landscape
Traditionally, compensation decisions have been made through a combination of market research, internal pay scales, and managerial discretion. HR departments typically rely on annual salary surveys, industry benchmarks, and company-specific policies to determine appropriate pay levels for various roles. This process often involves creating job descriptions, assigning them to pay grades, and establishing salary ranges for each grade.
However, this conventional approach has several inherent limitations. Firstly, it can be slow to respond to rapid market changes, potentially leaving organizations with outdated salary structures that fail to attract or retain top talent. Secondly, the reliance on historical data and human judgment can perpetuate existing pay inequities, particularly for underrepresented groups.
Moreover, traditional methods often struggle to account for the nuanced differences in individual contributions, skills, and potential. This can lead to a one-size-fits-all approach that fails to recognize and reward high performers adequately. Additionally, the complexity of modern job roles and the increasing importance of soft skills make it challenging to accurately value positions using traditional job evaluation methods.
Another significant challenge in the current landscape is the lack of transparency. Many organizations maintain a culture of pay secrecy, which can breed mistrust and perceptions of unfairness among employees. This lack of openness can also make it difficult for companies to identify and address pay disparities proactively.
How AI and Machine Learning Work in Compensation
Artificial Intelligence and machine learning are transforming the compensation landscape by introducing data-driven, objective decision-making processes. At its core, AI in compensation involves using algorithms to analyze vast amounts of data, identify patterns, and make predictions or recommendations based on these insights.
Machine learning, a subset of AI, enables these systems to improve their accuracy over time as they process more data. In the context of compensation, this means that AI systems can continuously refine their understanding of fair pay practices, market trends, and individual performance metrics.
The data used in AI-driven compensation systems comes from a variety of sources. Internal data may include employee demographics, performance reviews, skill assessments, tenure, and historical pay information. External data sources often encompass industry salary surveys, labor market statistics, economic indicators, and even social media sentiment analysis to gauge public perception of companies and roles.
Key algorithms employed in these systems include regression analysis for predicting salary trends, clustering algorithms for grouping similar job roles, and neural networks for complex pattern recognition in compensation data. Natural Language Processing (NLP) techniques are also used to analyze job descriptions and match them with appropriate pay scales.
One of the most powerful aspects of AI in compensation is its ability to consider multiple factors simultaneously. While a human analyst might struggle to balance ten or twenty variables, an AI system can easily process hundreds of data points to arrive at a fair and competitive compensation recommendation.
These systems can also identify non-obvious correlations that human analysts might miss. For example, an AI system might discover a link between certain employee benefits and improved retention rates, allowing companies to optimize their total rewards packages more effectively.
It’s important to note that while AI systems can provide powerful insights and recommendations, they are designed to augment human decision-making rather than replace it entirely. HR professionals and managers still play a crucial role in interpreting AI outputs, considering unique contextual factors, and making final decisions.
Issues AI Can Solve in Compensation
Eliminating Bias
One of the most significant advantages of AI-driven compensation systems is their potential to eliminate unconscious bias from pay decisions. Unlike humans, AI doesn’t have preconceived notions or personal preferences that can inadvertently influence decision-making.
These systems analyze objective data points such as skills, experience, performance metrics, and market rates without being swayed by factors like gender, race, age, or personal relationships. This data-driven approach helps ensure that compensation is based on merit and value to the organization rather than subjective impressions or unconscious biases.
AI can also identify patterns of bias in historical pay data. For instance, it might detect that employees from certain demographics have consistently received lower raises despite similar performance ratings. By flagging these discrepancies, AI enables organizations to address longstanding inequities that may have gone unnoticed.
Moreover, AI systems can be programmed to actively counteract known biases. For example, they can be designed to ignore names or other identifying information when making initial compensation recommendations, further reducing the potential for discrimination.
Pay Equity
AI excels at analyzing large datasets to identify pay gaps across various demographics. These systems can quickly compare compensation levels across gender, race, age, and other protected characteristics, controlling for factors like job level, experience, and performance.
By providing a comprehensive view of pay equity across the organization, AI enables HR teams to identify and address disparities proactively. This is particularly valuable for large organizations where manual analysis of pay equity would be time-consuming and prone to error.
AI systems can also simulate the impact of different pay adjustment scenarios. For instance, they can calculate the budget required to close identified pay gaps and suggest phased approaches to achieving pay equity over time.
Furthermore, AI can help organizations maintain pay equity on an ongoing basis. As new hires are made and employees receive promotions or transfers, the system can flag any decisions that might create new pay disparities, allowing HR to address these issues in real-time.
Market Rate Analysis
AI-driven compensation systems can perform real-time monitoring of industry compensation trends, providing organizations with up-to-date information on competitive pay rates. This capability is particularly valuable in fast-moving industries where salary expectations can change rapidly.
These systems can aggregate data from multiple sources, including salary surveys, job postings, and even social media discussions about compensation. By analyzing this diverse dataset, AI can provide a more comprehensive and nuanced view of market rates than traditional methods.
AI can also segment market data in sophisticated ways, allowing organizations to benchmark against the most relevant comparators. For instance, it might consider factors like company size, industry subsector, geographic location, and even the specific technologies or skills required for a role.
Perhaps most importantly, AI systems can predict future salary trends based on historical data and current market indicators. This predictive capability allows organizations to be proactive in their compensation strategies, adjusting pay scales in anticipation of market changes rather than reacting to them after the fact.
Performance-Based Pay
AI brings a new level of objectivity and sophistication to performance-based pay systems. By analyzing a wide range of performance metrics, these systems can create more nuanced and accurate assessments of employee contributions.
Traditional performance-based pay often relies heavily on manager ratings, which can be subjective and inconsistent. AI systems can complement these ratings with hard data on productivity, quality of work, customer satisfaction scores, and other relevant metrics. This multi-faceted approach provides a more holistic view of employee performance.
Moreover, AI can identify which performance metrics are most closely correlated with business success in different roles. This allows organizations to tailor their performance-based pay systems to incentivize the behaviors and outcomes that truly drive value.
AI systems can also personalize incentive structures based on individual motivations and career goals. By analyzing data on employee preferences and past performance, these systems can suggest tailored reward packages that are most likely to motivate each individual.
Retention Risk Assessment
AI-driven compensation systems can play a crucial role in identifying employees at risk of leaving due to compensation issues. By analyzing patterns in historical turnover data, these systems can identify the compensation-related factors that are most predictive of employee departures.
These systems can consider a wide range of variables, including an employee’s current pay relative to market rates, their pay trajectory over time, the competitiveness of their benefits package, and even non-monetary factors like work-life balance and career development opportunities.
AI can also predict when an employee might be approaching a “flight risk” threshold. For instance, it might identify that employees in certain roles tend to start looking for new opportunities after two years if they haven’t received a significant pay increase or promotion.
Armed with these insights, HR teams can take proactive steps to retain valuable employees. This might involve targeted salary adjustments, personalized career development plans, or changes to job responsibilities to increase engagement and satisfaction.
Practical Implementation of AI in Compensation
Data Collection and Preparation
The foundation of any AI-driven compensation system is high-quality, comprehensive data. Organizations need to collect and integrate data from various internal sources, including HRIS systems, performance management tools, and financial databases. This internal data should cover elements like employee demographics, job histories, performance ratings, and historical compensation information.
External data is equally important and may include industry salary surveys, labor market statistics, economic indicators, and competitor information. The challenge lies in ensuring that all this data is accurate, up-to-date, and formatted consistently for use by AI algorithms.
Data privacy is a critical consideration in this process. Organizations must ensure that they are collecting and using employee data in compliance with relevant regulations like GDPR or CCPA. This often involves anonymizing data, securing explicit consent for data use, and implementing robust data security measures.
It’s also important to address potential biases in historical data. If past compensation decisions were influenced by biases, simply feeding this data into an AI system could perpetuate these inequities. Organizations may need to clean or adjust historical data to ensure it doesn’t reinforce unfair practices.
Choosing the Right AI Tools
The market for AI-driven compensation tools is rapidly evolving, with options ranging from standalone solutions to modules within larger HR software suites. When selecting a tool, organizations should consider factors like the complexity of their compensation structure, the size of their workforce, and their specific goals for implementing AI in compensation.
Key features to look for include the ability to integrate with existing HR systems, robust data visualization capabilities, and transparent algorithms that can be audited for fairness. The tool should also be flexible enough to accommodate the organization’s unique compensation philosophy and practices.
It’s crucial to evaluate the track record of potential AI vendors. Look for case studies or references from organizations similar to yours. Consider the vendor’s approach to data security, their commitment to ongoing system improvements, and the level of support they offer during implementation and beyond.
Remember that no AI tool is perfect out of the box. Be prepared for a period of fine-tuning and customization to align the system with your organization’s specific needs and culture. The best tools will offer the flexibility to adjust algorithms and rules as your compensation strategy evolves.
Integration with Existing HR Systems
Successful implementation of AI in compensation requires seamless integration with existing HR systems. This typically involves connecting the AI tool with your HRIS, performance management system, and potentially financial systems.
The goal is to create a unified ecosystem where data flows automatically between systems. For example, when an employee receives a promotion in the HRIS, this should trigger an automatic review of their compensation in the AI system. Similarly, performance data should feed directly into compensation recommendations.
This integration often requires careful planning and collaboration between HR, IT, and the AI vendor. It’s important to map out data flows, identify potential integration challenges, and establish protocols for data synchronization and error handling.
Consider implementing a pilot or phased rollout to test integrations and workflows before full implementation. This allows you to identify and address any issues on a smaller scale, reducing risk and ensuring a smoother full-scale deployment.
Training and Change Management
Implementing AI in compensation represents a significant change for HR professionals and managers. It’s crucial to provide comprehensive training on how to use the new tools effectively and interpret their outputs.
This training should cover not just the technical aspects of using the AI system, but also the underlying principles of how it works. Understanding the basics of the algorithms and data sources used can help build trust in the system’s recommendations.
Change management is equally important. Communicate clearly with all stakeholders about why the organization is implementing AI in compensation, what benefits it will bring, and how it will impact current processes. Address concerns proactively, particularly around job security and the role of human judgment in compensation decisions.
Consider appointing “AI champions” within the HR team who can serve as go-to resources for questions and support during and after implementation. These individuals can help bridge the gap between the technical aspects of the AI system and its practical application in day-to-day HR operations.
Continuous Monitoring and Adjustment
Implementing AI in compensation is not a one-time event, but an ongoing process of refinement and optimization. Regular audits of the AI system’s recommendations are crucial to ensure it continues to align with the organization’s compensation philosophy and produces fair, competitive outcomes.
These audits should look for any unexpected patterns or biases in the AI’s outputs. For example, are certain groups consistently receiving lower compensation recommendations despite similar qualifications and performance? Are the system’s market rate predictions aligning with real-world observations?
Based on these audits and real-world outcomes, be prepared to refine the AI algorithms over time. This might involve adjusting the weighting of different factors, incorporating new data sources, or tweaking decision rules to better reflect your organization’s priorities.
It’s also important to stay abreast of developments in AI technology and compensation best practices. As new techniques emerge, consider how they might be incorporated to improve your system’s accuracy and fairness.
Conclusion
AI-driven compensation represents a significant leap forward in the pursuit of fair, competitive, and strategic pay practices. By leveraging the power of data and advanced algorithms, organizations can address longstanding challenges in compensation management, from eliminating bias to ensuring pay equity and optimizing performance-based rewards.
The benefits of implementing AI in compensation are clear: more objective decision-making, improved pay equity, better alignment with market rates, and the ability to proactively address retention risks. However, successful implementation requires careful planning, robust data management, and a commitment to ongoing refinement and oversight.
As we look to the future, it’s clear that AI will play an increasingly central role in compensation management. Organizations that embrace this technology thoughtfully and strategically will be well-positioned to attract, retain, and motivate top talent in an increasingly competitive labor market.
For HR professionals, now is the time to explore AI-driven compensation solutions and consider how they might be leveraged to enhance your organization’s compensation strategy. By combining the power of AI with human expertise and judgment, we can create compensation systems that are not only more efficient but also more fair, transparent, and aligned with organizational goals.
Further Reading and Sources
- “Artificial Intelligence for HR: Use AI to Support and Develop a Successful Workforce” by Ben Eubanks
- “The Future of Work: Attract New Talent, Build Better Leaders, and Create a Competitive Organization” by Jacob Morgan
- WorldatWork’s “Compensation Technology Briefing” series
- MIT Sloan Management Review’s articles on AI in HR
- Harvard Business Review’s collection on AI and Machine Learning